Question 5 5.1. A study was conducted to ascertain the effectiveness of a treatment of diseased plants. A recorded value of Y = 1 indicates that the treatment is effective and a value of Y=0 is recorded when no effect is noticed. The outcome of the effectiveness of the treatment considers the treatment dosage, the above ground biomass, the rate of growth and the height of a plant recorded as I if above average height and recorded 0 as below average height. The following partial data set is provided. EFFECTS (Y) DOSAGE X₁ 0.2138 0.3907 0.2138 1 1 0 (Intercept) HEIGHT BIOMASS RATE BIOMASS X₂ RATEX, HEIGHT X₁ 0.0525 0 0.0264 0.0920 The output for running the full logistic regression model is given below: Coefficients Estimate -1.23 0.31 0.33 0.34 1.2 1.3 Std. Error 0.6795 0.6821 5.9374 24112 DU z value -1.653 0.456 2.594 3.167 Pr>Z) 0.0984 0.6484 0.0095 0.0076 CARE
Question 5 5.1. A study was conducted to ascertain the effectiveness of a treatment of diseased plants. A recorded value of Y = 1 indicates that the treatment is effective and a value of Y=0 is recorded when no effect is noticed. The outcome of the effectiveness of the treatment considers the treatment dosage, the above ground biomass, the rate of growth and the height of a plant recorded as I if above average height and recorded 0 as below average height. The following partial data set is provided. EFFECTS (Y) DOSAGE X₁ 0.2138 0.3907 0.2138 1 1 0 (Intercept) HEIGHT BIOMASS RATE BIOMASS X₂ RATEX, HEIGHT X₁ 0.0525 0 0.0264 0.0920 The output for running the full logistic regression model is given below: Coefficients Estimate -1.23 0.31 0.33 0.34 1.2 1.3 Std. Error 0.6795 0.6821 5.9374 24112 DU z value -1.653 0.456 2.594 3.167 Pr>Z) 0.0984 0.6484 0.0095 0.0076 CARE
Chapter6: Exponential And Logarithmic Functions
Section6.8: Fitting Exponential Models To Data
Problem 3TI: Table 6 shows the population, in thousands, of harbor seals in the Wadden Sea over the years 1997 to...
Related questions
Question

Transcribed Image Text:Question 5
5.1. A study was conducted to ascertain the effectiveness of a treatment of diseased plants. A recorded
value of Y = 1 indicates that the treatment is effective and a value of Y = 0 is recorded when no
effect is noticed. The outcome of the effectiveness of the treatment considers the treatment
dosage, the above ground biomass, the rate of growth and the height of a plant recorded as 1 if
above average height and recorded 0 as below average height. The following partial data set is
provided.
EFFECTS (Y) DOSAGE X₁
0.2138
0.3907
0.2138
1
1
0
The output for running the full logistic regression model is given below:
Coefficients
(Intercept)
HEIGHT
BIOMASS
RATE
DOSAGE
Estimate
-1.23
0.31
0.33
0.34
-1.86
BIOMASS X₂ RATE X₂
0.0525
1.1
0.0264
1.2
0.0920
1.3
Estimate
-1.25
0.21
Std. Error
0.6795
0.6821
5.9374
2.4112
2.1420
Log Likelihood: -2.579
The output for the reduced model is given below:
Coefficients
(Intercept)
BIOMASS
Std. Error
0.4129
4.7390
6
z value
-1.653
0.456
2.594
3.167
-0866
z value
-2.488
2.572
HEIGHT X₂
0
1
1
Pr(>Z)
0.0984
0.6484
0.0095
0.0076
0.3864
Pr(>Z)
0.0144
0.0101

Transcribed Image Text:RATE
0.41
Log Likelihood: -4.246
Answer the following questions.
2.4223
5.1.1. State the full and reduced logistic regression models.
5.1.2. Give a complete and formal test to establish whether or not predictors HEIGHT and
DOSAGE are necessary in the model. Test at a = 0.05.
3.161
5.2. Give the estimated logistic regression decided upon and give the odds ratios for regression
coefficients b₂and by of this regression.
5.3. Use the estimated logistic regression from Question 5.2 to find the probability of the treatment
being effective if the amount of biomass above ground is 3 grams and the rate of growth is 1.3.
Interpret the result.
True Classification
Y=0
Y = 1
5.4. To establish whether RATE is indeed needed to predict the effectiveness of a treatment, a formal
test can be used. Give the name of this test, state the hypothesis, test statistic formula and the
decision rule.
0.0076
5.5. Logistic regression's power lies also in the ability to predict a new observation's class. In order to
do that, a cut-off point for the different classes must be established. In this study, an effective
treatment of a diseased plant was recorded as (Y = 1) and if not, it was recorded as (Y = 0).
The following table shows the predictions by the classification method.
Y = 0
45
10
Predicted
Y = 1
17
28
Total
62
38
5.5.1. The above table was created based on the following rule: Predict 1 if ft ≥ 0.6 and predict
0 if ft < 0.6, explain this rule.
5.5.2. Discuss the above table. Also give the error prediction rate.
5.5.3. Give the sensitivity and 1- specificity values.
5.5.4. What are the motivations for using a receiver operating characteristic called a ROC curve
and the AUC, area under the curve, in classification? Would you consider the
classification based on the rule in Question 5.5.1 as good enough based on the values in
Question 5.5.3?
Expert Solution

This question has been solved!
Explore an expertly crafted, step-by-step solution for a thorough understanding of key concepts.
Step by step
Solved in 5 steps with 6 images

Recommended textbooks for you
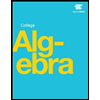
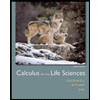
Calculus For The Life Sciences
Calculus
ISBN:
9780321964038
Author:
GREENWELL, Raymond N., RITCHEY, Nathan P., Lial, Margaret L.
Publisher:
Pearson Addison Wesley,
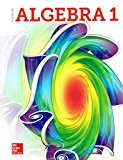
Glencoe Algebra 1, Student Edition, 9780079039897…
Algebra
ISBN:
9780079039897
Author:
Carter
Publisher:
McGraw Hill
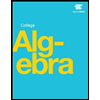
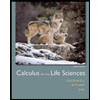
Calculus For The Life Sciences
Calculus
ISBN:
9780321964038
Author:
GREENWELL, Raymond N., RITCHEY, Nathan P., Lial, Margaret L.
Publisher:
Pearson Addison Wesley,
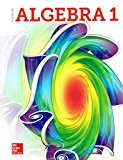
Glencoe Algebra 1, Student Edition, 9780079039897…
Algebra
ISBN:
9780079039897
Author:
Carter
Publisher:
McGraw Hill
Algebra & Trigonometry with Analytic Geometry
Algebra
ISBN:
9781133382119
Author:
Swokowski
Publisher:
Cengage
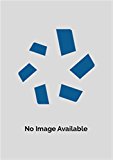
Big Ideas Math A Bridge To Success Algebra 1: Stu…
Algebra
ISBN:
9781680331141
Author:
HOUGHTON MIFFLIN HARCOURT
Publisher:
Houghton Mifflin Harcourt