Please answer each of the following questions in detail and provide examples for better clarity, wherever applicable. Provide in-text citations. Figure 1 shows a typical composition of neurons in a net. This discussion questions aimed at learning how natural composition of neural nets can be mimicked to derive algorithms that can provide prediction at higher levels of complexity. Figure 1 is attached (Figure 1 : Typical Composition of Neurons in a Net) Consider a multiple regression model of your choice containing two predictors. Calculate the values of the regression equation with a range chosen by you for the values of the predictors. Provide the graph of the regression equation which is a plane. Augment your regression model by a sigmoid posterior filter. Calculate the values of the regression equation within the range chosen in part 1. Provide the graph of the regression equation, which will be a sigmoidal surface. Show that this graph cannot have a local extremum. Now, add another regression model with sigmoid posterior containing the same two predictors in the previous part. Repeat parts 1 and 2 for this new regression model. Now consider a model of a neural net, which has two parallel hidden layers, which are the two regression models considered above. The output of the net is simply the superposition of the two regression models. Show that by proper choice of the parameters you can have an output, which has a local extremum within the choice for the range of the values of the predictor. Provide the graph of the regression equation. How does this observation indicate an application of neural networks modeling in practice?
Please answer each of the following questions in detail and provide examples for better clarity, wherever applicable. Provide in-text citations. Figure 1 shows a typical composition of neurons in a net. This discussion questions aimed at learning how natural composition of neural nets can be mimicked to derive algorithms that can provide prediction at higher levels of complexity. Figure 1 is attached (Figure 1 : Typical Composition of Neurons in a Net) Consider a multiple regression model of your choice containing two predictors. Calculate the values of the regression equation with a range chosen by you for the values of the predictors. Provide the graph of the regression equation which is a plane. Augment your regression model by a sigmoid posterior filter. Calculate the values of the regression equation within the range chosen in part 1. Provide the graph of the regression equation, which will be a sigmoidal surface. Show that this graph cannot have a local extremum. Now, add another regression model with sigmoid posterior containing the same two predictors in the previous part. Repeat parts 1 and 2 for this new regression model. Now consider a model of a neural net, which has two parallel hidden layers, which are the two regression models considered above. The output of the net is simply the superposition of the two regression models. Show that by proper choice of the parameters you can have an output, which has a local extremum within the choice for the range of the values of the predictor. Provide the graph of the regression equation. How does this observation indicate an application of neural networks modeling in practice?
Chapter9: Computer Networks
Section: Chapter Questions
Problem 3VE
Related questions
Question
Please answer each of the following questions in detail and provide examples for better clarity, wherever applicable. Provide in-text citations.
Figure 1 shows a typical composition of neurons in a net. This discussion questions aimed at learning how natural composition of neural nets can be mimicked to derive
Figure 1 is attached
(Figure 1 : Typical Composition of Neurons in a Net)
- Consider a multiple regression model of your choice containing two predictors. Calculate the values of the regression equation with a range chosen by you for the values of the predictors. Provide the graph of the regression equation which is a plane.
- Augment your regression model by a sigmoid posterior filter. Calculate the values of the regression equation within the range chosen in part 1. Provide the graph of the regression equation, which will be a sigmoidal surface. Show that this graph cannot have a local extremum.
- Now, add another regression model with sigmoid posterior containing the same two predictors in the previous part. Repeat parts 1 and 2 for this new regression model.
- Now consider a model of a neural net, which has two parallel hidden layers, which are the two regression models considered above. The output of the net is simply the superposition of the two regression models. Show that by proper choice of the parameters you can have an output, which has a local extremum within the choice for the range of the values of the predictor. Provide the graph of the regression equation.
- How does this observation indicate an application of neural networks modeling in practice?

Transcribed Image Text:Input Layer
Hidden Layer
Output Layer
4 = h10 + hX1+ h12X2
+ ... + hkXK
eh -1
H(l)=
%3D
el +1
X1
L = Bo + BH,(1,) + B2H2(l2)
+ .. + BmHmlm)
2= h20 + h21x1 + h22x2
+ .-- + hakXk
ek -1
if response
variable is
1
...
g(L) =
X2
1+el
qualitative.
H2(l2) =
ek+ 1
if response
variable is
quantitative.
Im = hmo + hmix1 + hm2X2
+ - + hmkXk
%3D
...
elm – 1
Hm(m) =
%3D
elm +1
...
Expert Solution

This question has been solved!
Explore an expertly crafted, step-by-step solution for a thorough understanding of key concepts.
This is a popular solution!
Trending now
This is a popular solution!
Step by step
Solved in 2 steps

Knowledge Booster
Learn more about
Need a deep-dive on the concept behind this application? Look no further. Learn more about this topic, computer-science and related others by exploring similar questions and additional content below.Recommended textbooks for you
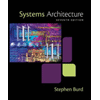
Systems Architecture
Computer Science
ISBN:
9781305080195
Author:
Stephen D. Burd
Publisher:
Cengage Learning
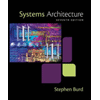
Systems Architecture
Computer Science
ISBN:
9781305080195
Author:
Stephen D. Burd
Publisher:
Cengage Learning